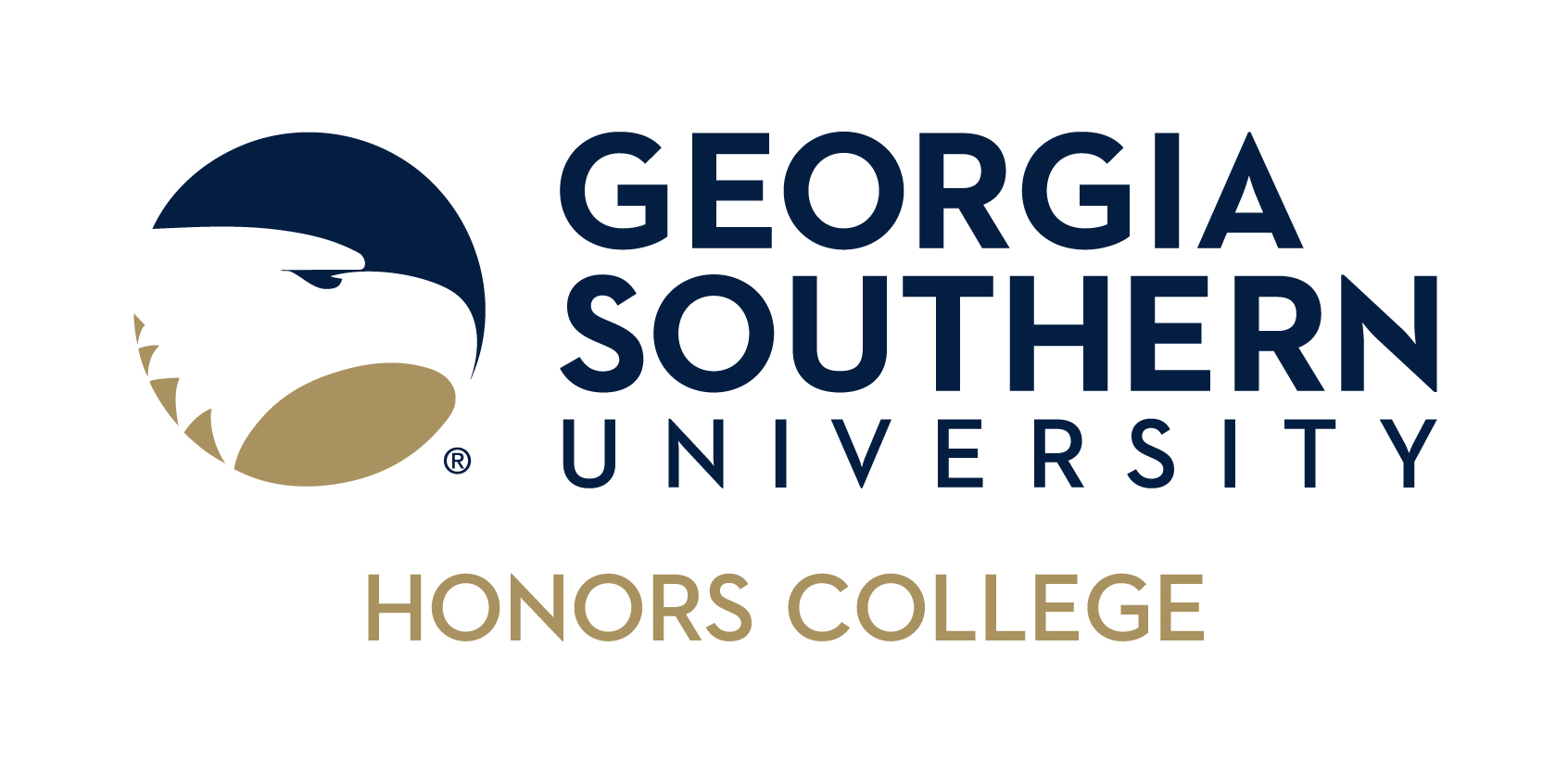
Honors College Theses
Publication Date
4-6-2023
Major
Computer Science (B.S.)
Document Type and Release Option
Thesis (open access)
Faculty Mentor
Lixin Li
Abstract
Fine particulate matter or PM2.5 can be described as a pollution particle that has a diameter of 2.5 micrometers or smaller. These pollution particle values are measured by monitoring sites installed across the United States throughout the year. While these values are helpful, a lot of areas are not accounted for as scientists are not able to measure all of the United States. Some of these unmeasured regions could be reaching high PM2.5 values over time without being aware of it. These high values can be dangerous by causing or worsening health conditions, such as cardiovascular and lung diseases. Within this study, fine particulate matter values were interpolated at centroids of all counties in the United States throughout 2009 using the Python programming language and a spatiotemporal Inverse Distance Weighting (IDW) interpolation method. Machine learning concepts, such as ten-fold cross-validation, and error statistics were used to assess the accuracy of the estimated PM2.5 values. The created Python programs display a graphical user interface for easy interaction between a user and the system. This allows the system to be used by more than just experts. The values reported in this study can also be used to determine if unmeasured county areas are reaching unsafe PM2.5 values throughout the year.
Recommended Citation
Entrekin, Kelly M., "A Graphical User Interface using Spatiotemporal Interpolation to determine Fine Particulate Matter Values in the United States" (2023). Honors College Theses. 837.
https://digitalcommons.georgiasouthern.edu/honors-theses/837
Included in
Algebra Commons, Applied Statistics Commons, Environmental Health and Protection Commons, Environmental Monitoring Commons, Environmental Studies Commons, Numerical Analysis and Computation Commons, Numerical Analysis and Scientific Computing Commons, Other Computer Sciences Commons, Programming Languages and Compilers Commons, Science and Technology Studies Commons, Spatial Science Commons