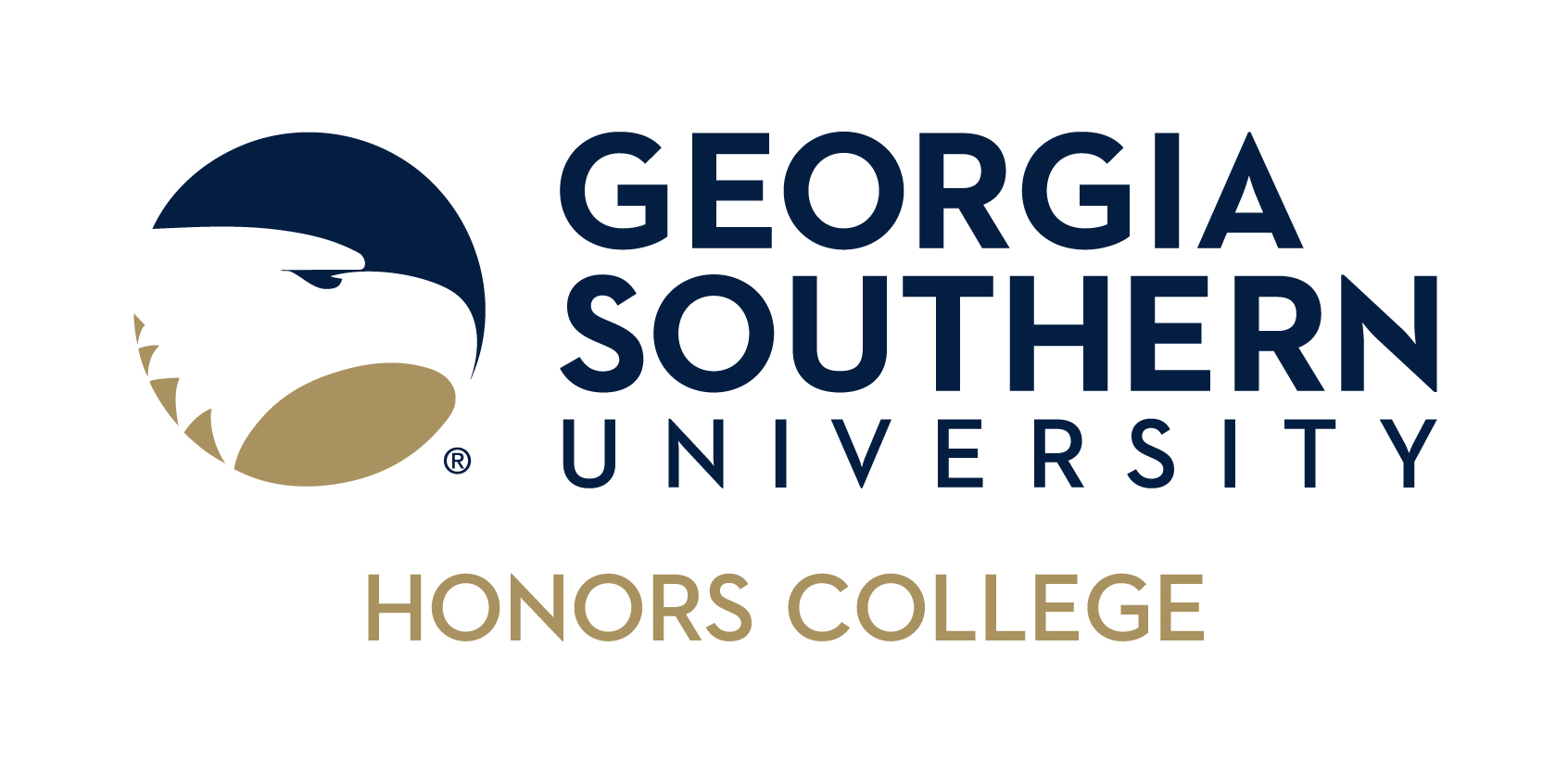
Honors College Theses
Publication Date
2016
Major
Electrical Engineering (B.S.)
Document Type and Release Option
Thesis (open access)
Faculty Mentor
Dr. Rami Haddad
Abstract
In this work, we propose the use of non-linear, autoregressive neural network models for predicting video frame sizes. This model utilizes H.265 encoded video traces as inputs and the predicted future frame sizes as outputs. This model is developed to predict ultra-high definition video frame encoded with H.265 within IP networks. The video I, P, and B frames are predicted separately to improve model prediction accuracy. This approach is verified in MATLAB using various H.265 video traces. The results indicate that the proposed models were able to predict the video traffic fairly accurately.
Recommended Citation
Daly, Collin, "Novel H.265 Video Traffic Prediction Models Using Artificial Neural Networks" (2016). Honors College Theses. 197.
https://digitalcommons.georgiasouthern.edu/honors-theses/197
Included in
Digital Communications and Networking Commons, Electrical and Computer Engineering Commons