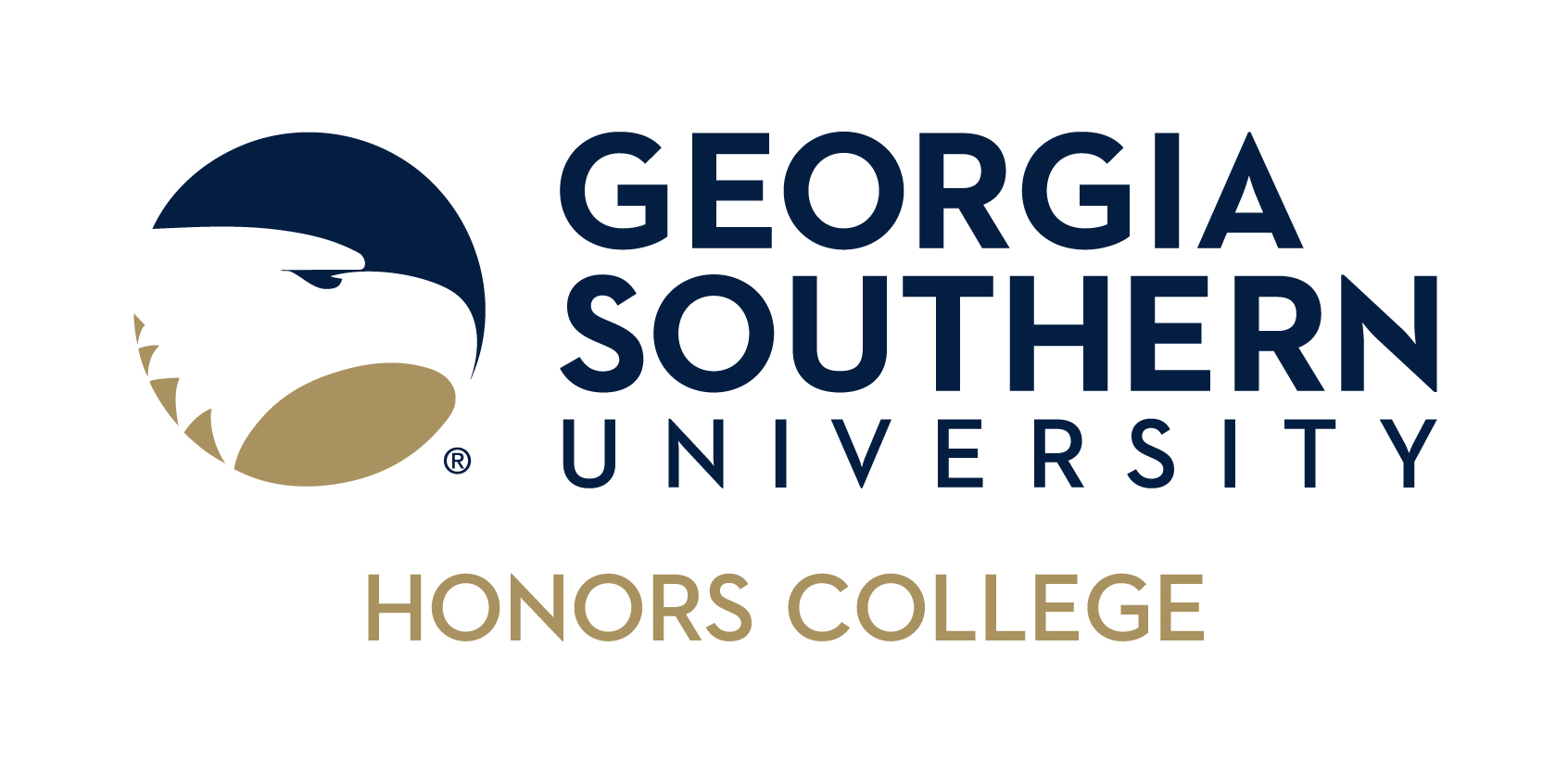
Honors College Theses
Publication Date
5-2-2023
Major
Mechanical Engineering (B.S.)
Document Type and Release Option
Thesis (open access)
Faculty Mentor
Dr. Hossain Ahmed
Abstract
The ability to predict material behavior that undergoes various loading conditions is critical to the development of reliable and safe components. Thermal and mechanical fatigue loading can cause significant damage to materials, leading to failure and potential safety hazards. Machine learning algorithms have emerged as a promising tool for improving accuracy and efficiency of predicting material behavior under such loading conditions. This research provides a comprehensive overview of a machine learning algorithm that is able to analyze and predict material state independently of the loading sequence. Unidirectional carbon fiber reinforced polymer (UD CFRP) composite which has undergone two different loading sequences is analyzed. On one hand, the pristine material sustains 40 cycles of thermal fatigue followed by a break where data is acquired and then the specimen undergoes 150k cycles of mechanical fatigue. The second load sequence consists of the same cycles but in a reversed manner. Data collected from a small section of the composite in each stage was used to train a Naive Bayes classifier. A feature selection process is carried out with the use of principal component analysis to identify the most relevant parameters for use.The results show that the Naive Bayes classifier can accurately predict the fatigue sequence of UD CFRP samples under thermal and mechanical loading conditions with an accuracy of up to 80%. The findings of this paper can have significant implications for the analysis of structures subjected to thermal and fatigue loading.
Recommended Citation
Abadie, Ana B., "Utilization of Machine Learning to Investigate Material State" (2023). Honors College Theses. 876.
https://digitalcommons.georgiasouthern.edu/honors-theses/876